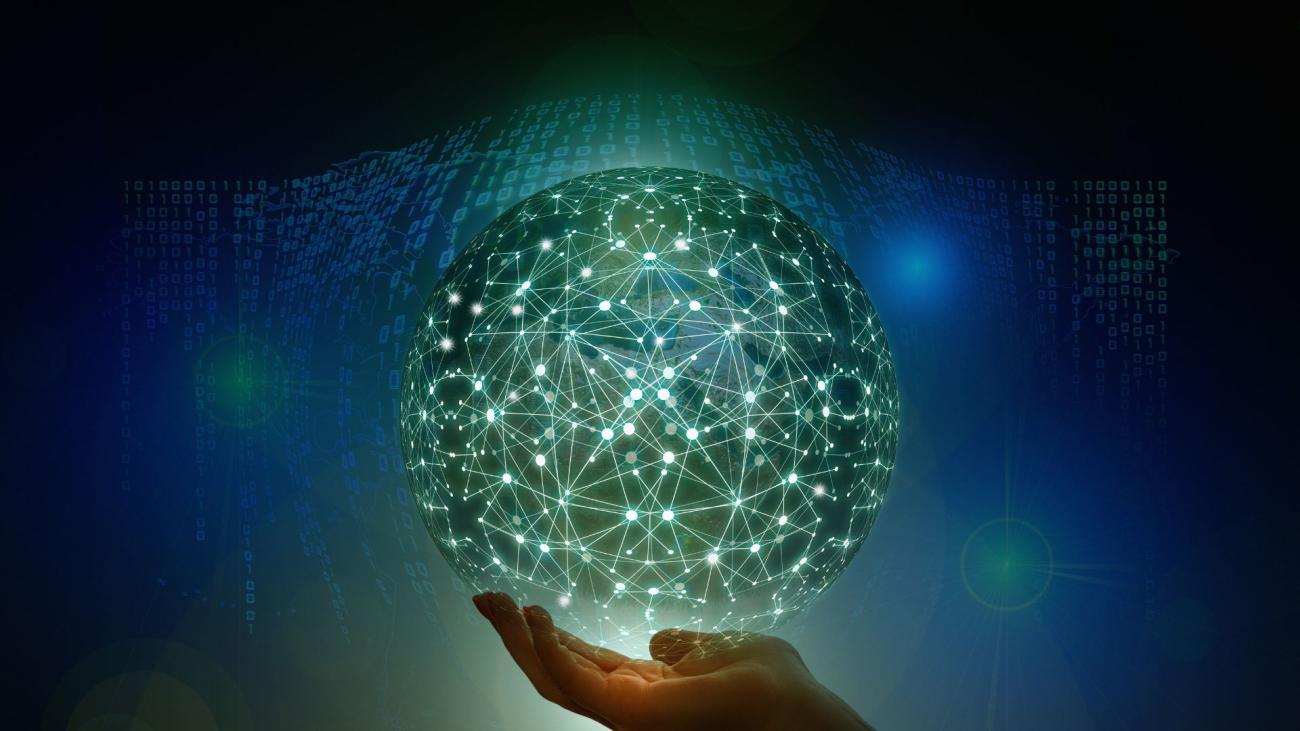
We are awash with data. Eighty percent of that data is unstructured, and that number is growing between 55 and 65% annually. Case in point, in 2022, 500 hours of video content were uploaded to YouTube every minute. Much of this unstructured data is text or “natural language” data, and accounts for approximately three-quarters of all recorded digital data. “Text” includes, but is by no means limited to, websites, blogs, social media posts, research papers, news articles, and transcripts. In this age of AI, that text also includes what Generative AI tools (rather than human authors) compose.
By its very nature, unstructured data is difficult to search or analyze, and a time-consuming task to parse in its entirety. New technological methods such as Computational Text Analysis (CTA), an umbrella term for various digital tools and quantitative techniques that optimize the power of computers and software, use a method called, “distant reading” or “text mining.” With CTA, Dartmouth researchers can gather vast amounts of unstructured text and examine it all at once, when previously they were limited to “close reading” a single or handful of texts at a time.
Head of Research Facilitation at Dartmouth Libraries, Lora Leligdon, shares that text analysis enables researchers to go beyond individual texts, revealing meaningful trends across vast datasets while connecting scholars across disciplines. When we pair close reading and text mining, we can develop new insights, make different interpretations, and ask new kinds of questions.